Finding a Triple Slash for Pitchers
Determining the three most descriptive stats of a starting pitcher’s success
Back in February, I read an article from Ben Clemens, and then a follow-up from Eli Ben-Porat. They debated just how much AVG adds to the triple slash, given you already have the other two elements. Clemens essentially said “not much”, and Ben-Porat pointed out that AVG is the foundation of OBP and SLG to a large extent, and proposed BAPP as an alternative to AVG/OBP/SLG.
What struck me most from this was:
a) the amount of overlap between each of the three stats and
b) how much variation in runs scored can be explained by these three stats alone.
Recently, I’ve been working on a set of questions regarding starting pitching -- how much starters tweak their repertoire over time, how much impact that has, and just how neatly I can summarize the effectiveness of a particular start. I decided a good place to start would be working backwards and determining which stats are most closely tied to run prevention over time.
A New Triple Slash: WHIP/LOB%/HR per 9
To do this, I followed a similar methodology to Clemens and Ben-Porat, but instead of team-level stats, I decided to look at individual pitcher’s seasons. I feel there’s nuance in how a pitcher goes about his business, and I didn’t want to smooth it over by aggregating by team. As it turns out, I later ran the same analysis on the team level, and got similar results.
So, I pulled all qualified starting pitcher seasons from 2010-2023, excluding 2020. This left me with 937 individual seasons to evaluate, and 495 since 2015 (introduction of Statcast). I may repeat this exercise to see if relief pitchers are any different.
I measured the effectiveness of a pitcher by ER/700 PA, since that roughly correlates to 162 IP. Basically asking: if a starter faced enough batters to qualify, how many runs can we expect him to give up?
I then tested a bunch of different variables to see what was most heavily correlated to ER/700 PA. These included: AVG, WHIP, K/9, K%, BB/9, BB%, Barrel%, HardHit%, LOB%, HR/9, BABIP, Zone%, O-Swing%, Z-Swing%.
You can see the R^2 values of each of these variables below:
WHIP, unsurprisingly, came in as a strong variable, and slightly outperformed AVG, presumably since it also accounts for traffic on the basepaths in the form of walks.
WHIP, R^2 = 0.53
HR per 9, R^2 = 0.42
Also influential was a pitcher’s HR/9 rate, capturing the extent to which he avoids (or in the case of 2018 Dylan Bundy, does not avoid) the long ball.
LOB%, R^2 = 0.59
However, the most influential statistic by correlation to ER/700 PA was LOB%, the percentage of baserunners a pitcher stranded over the course of the season. It displayed a pretty strong (r = -0.77) negative correlation to ER allowed, and as a result clocked in with a 0.59 r^2 value, the strongest of any of the stats I tested.
This makes sense from the point of view that LOB% answers the question of: when a pitcher is in danger of having baserunners come around, how effectively does he prevent this from happening? It does, however, raise a few questions as well.
The Problem with LOB%
Now, this initial analysis left me with a triple slash of WHIP/LOB%/HR per 9. Here’s what I liked and what I didn’t like in terms of these stats.
The Pros: each of these stats, for the most part, tells me something different about the pitcher at hand.
WHIP describes the amount of traffic on the base paths. LOB% describes how often that traffic comes around to score. And HR/9 describes how often that processes is accelerated via the home run.
The Cons: LOB% is, for the most part, not a very consistent measure. It’s hard to discern how much of LOB% comes from actual skill -- for instance, striking batters out -- and how much comes from the sequence of the hits and outs recorded.
The question of ‘clutchness’ has been raging for years, and it’s unclear how much control over the order of events a pitcher has, let alone all the other aspects of pitching, including holding runners on, fielding his position, and situationally inducing ground balls.
To better understand the nature of each of the included stats, I investigated to see how often a pitcher’s best season by ER/700 PA matched up with their best season in each of the following categories. Of the 344 individual pitchers from 2010-2023, excluding 2020, here’s how often they matched up.
As you can see, the best season by ER/700 PA in a pitcher’s career matched their best season by WHIP 77% of the time, their best season by LOB 78% of the time, and their best season by HR/9 73% of the time. It matched all three 52% of the time.
This shows that an exceptional season by LOB% is heavily linked to an exceptional season overall, but not significantly more so than WHIP of HR/9. Of the best seasons included in this dataset (think 2018 deGrom, 2019 Cole, 2015 Grienke), almost all had exceptionally high LOB%. It’s not a necessity to have a great year, but it sure helps.
So, I decided to take a look at how ‘sticky’ each of these stats are by asking how correlated each stat is year over year. For instance, I correlated a pitcher’s WHIP from his 2010 season to his 2011 season, then again from 2011 to 2012, and so on.
The chart below can be read as follows: the correlation between WHIP in 2010 and WHIP in 2011 for the same pitcher is 0.465. The correlation for LOB% in 2010 and LOB% in 2011 for the same pitcher is 0.124. For HR/9 it is 0.358.
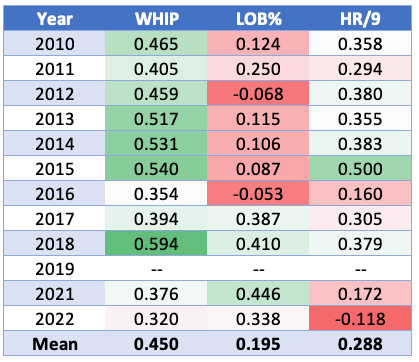
Clearly, WHIP is, on average, a much more consistent statistic than HR/9, and especially when compared to LOB%.
A pitcher likely has more control of WHIP, and LOB% may be attributed more as an artifact of regression to the mean than a true barometer of skill.
Building a Model
That said, I did want to use the descriptive power of WHIP, LOB%, and HR/9 to determine just how well they can explain a pitcher’s season. To do this, I built a multivariable linear regression with each of the three variables, and used them to predict a pitcher’s ER/700 PA for each year 2010-2023 (again excluding 2020).
Here’s what the model looked like:
Overall, the combined model came in with an R^2 value of 0.934, indicating that just WHIP, HR/9, and LOB% explain roughly 93% of a pitcher’s variance in ER/700 PA.
For context, Clemens found that a team’s triple slash explains 89.8% of variation in runs scored. So we are a little bit higher here, likely due to the fact that each stat provides a different look at a pitcher’s performance.
Overall, these results follow what we discussed above.
The best way to reduce your runs allowed is to prevent men on base from scoring, but that is also the least reliable measure included year-to-year. With a baseline of a similar WHIP and HR/9, two pitchers with widely variable LOB% rates will likely see that reflected in the runs they allow.
LOB% does a good amount of the talking today, it just won’t tell you much in terms of how lucky a pitcher will be tomorrow (or next year).
And here’s what a scatter plot of actual vs. predicted ER/700 PA values looked like:
Takeaways
The triple slash isn’t cut and dry for pitchers. There’s a whole lot of variability, even when working with relatively large sample sizes.
However, with just a few stats, you can predict the ability of a pitcher to a really strong degree. Even better than even a triple slash for a batter.
That said, this analysis probably doesn’t translate in terms of what happens on a game-by-game level. WHIP, LOB% and HR/9 are the building blocks of the tower of runs a pitcher gives up over a season, but I want to get a better look at the machinery on a smaller sample size. For those purposes, I’d want to employ more reliable Statcast metrics like quality of contact stats, which have some predictive value, as opposed to the descriptive stats I’ve included here.
Thanks for reading. Please let me know if there’s anything I missed or ways for me to improve in the future. I look forward to posting more on this topic and others coming soon.
Works Cited
Clemens, B. (2023) Triple-Slash Line Conundrum: Voros McCracken Edition, FanGraphs Baseball. Available at: https://blogs.fangraphs.com/triple-slash-line-conundrum-voros-mccracken-edition/ (Accessed: 28 July 2023).
Ben-Porat, E. (2023) The boomers were right, Porat. Available at: https://elibenporat.substack.com/p/the-boomers-were-right (Accessed: 28 July 2023).
When does past ERA become more predictive of future ERA than past FIP? (2023) Tangotiger blog. Available at: http://tangotiger.com/index.php/site/comments/when-does-past-era-become-more-predictive-of-future-era-than-past-fip (Accessed: 28 July 2023).